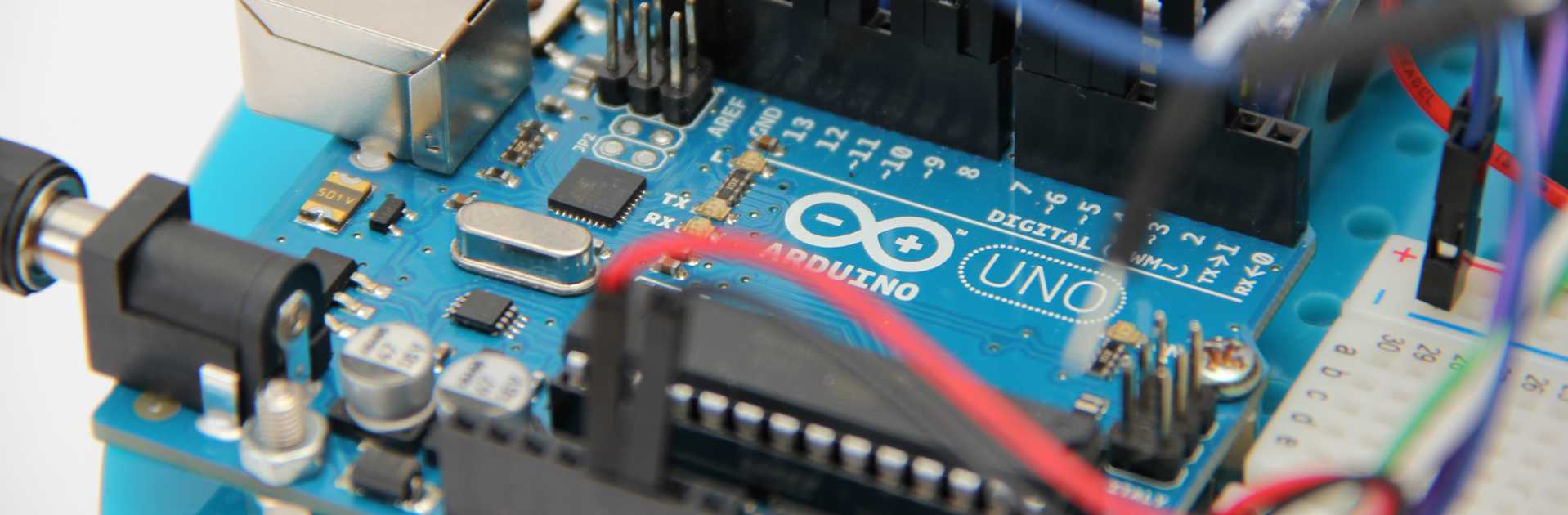
Research into cybernetic systems for estimation, decision-making and control under uncertainty for simple as well as complex real systems includes the system theory, system identification, parameter estimation, design of linear as well as nonlinear filters for state estimation, decision making, fault and change detection in dynamic systems, optimal control, adaptive control and adaptive signal processing in order to ensure higher adaptability, efficiency, quality and stability of cybernetic systems being designed.
Our research team specializes in the following key areas:
- Nonlinear filtering
- Fault detection
- Information fusion
- Adaptive dual control
We aim to develop new approaches and new formulations and find unification of problems and their solutions in these areas. We are mainly motivated by the fact that in order to obtain a high-quality and detailed description of real problems and real systems, nonlinear models and uncertainty – reflecting inaccuracies in measurements and fault behaviour – must be employed in order to allow for better alignment between models and reality than linear and deterministic models can provide.
Theoretical and Practical Use:
Outcomes of the research into unknown variable estimation can be used broadly in various fields of human activities; in particular, these include modelling of technical as well as non-technical processes, aviation industry, navigation, land transportation, health industry, economics, etc. In aviation industry and navigation, for example, these include algorithms designed to estimate position, velocity and attitude of vehicles and satellites. By taking uncertainty into consideration, we can find new solutions delivering higher quality. Results of research into fault and change detection include algorithms designed to detect either changes in systems behaviour, or system faults, as the case may be. These algorithms are employed to monitor, identify and control various systems, e.g. systems for modern buildings (heating, ventilation and air-conditioning) etc. Results of research into optimal and adaptive control include algorithms designed to ensure good quality of system identification and control. Adaptive algorithms make it possible to control systems containing not only unknown parameters, but also an unknown structure. In the field of information fusion research, our IDM research group designs methods for efficient treatment of multi-source data (e.g. different types of built-in sensors in devices). The fusion of data and estimates allows for decentralized information processing and enables identification and control in the event the centralized approach is not viable.
Research and Development Objectives in Basic Research:
Research and Development into Identification of Stochastic Dynamic Systems
Research activities are focused on systems providing estimates in form of probability densities of state under measurement, and systems that provide point estimates of unknown variables. Our effort is mainly focused on designing estimation systems to improve the quality of estimates, or to reduce computing complexity.
Research and Development into Detection of Changes and Faults of Monitored Systems
The challenge in this area is the systematic research into design of a new generation of detectors, providing not only decisions about system changes or faults, but also generating signals probing the monitored system in order to increase the quality and speed of detection.
Research into Control Systems under Uncertainty
The fundamental property of control system synthesis is the design of controls providing for optimal balancing of the system model, while maintaining the desired characteristic of the controlled system.
The team demonstrates more than twenty years of experience in this field, having participated in numerous scientific and application projects.
The key theoretical results achieved by the team include new approaches and methods in the field of local and global nonlinear filtering, nonlinear identification, predictive and bi-criterial dual control and passive and active detection; these results were published namely in renowned global journals with a high impact factor (Automatica, IEEE Transactions on Automatic Control, IEEE Transactions on Aerospace and Electronic Systems, Signal Processing), at congresses and symposia of the International Federation of Automatic Control – IFAC, and renowned conferences in the USA (Conference on Decision and Control, American Control Conference).
The team is also engaged in the development of a complex software package to implement a number of state estimation algorithms. The package makes it possible to describe the entire estimation problem, i.e. the actual data generator, its model and estimation method. Based on results hereby obtained, it can provide a complex evaluation of estimation quality, including performance comparison of individual methods.